Signalverarbeitung mit MATLAB und Künstlicher Intelligenz: Unterschied zwischen den Versionen
Zeile 18: | Zeile 18: | ||
== Introductiong == | == Introductiong == | ||
Signal processing has traditionally focused on analyzing, filtering, and interpreting signals in both the time and frequency domains. With the integration of AI, especially deep learning, the possibilities expand to include noise reduction and more. It is essential in many modern applications such as communications, audio and image processing, biomedical signal analysis, and control systems. | |||
Traditionally, classical algorithms have been taught to solve engineering problems. This project solves teaching problems using artificial intelligence and compares these classical algorithms. | Traditionally, classical algorithms have been taught to solve engineering problems. This project solves teaching problems using artificial intelligence and compares these classical algorithms. | ||
=== ''Signal Processing'' === | === ''Signal Processing Fundamentals'' === | ||
==== | ==== Time Domain vs. Frequency Domain==== | ||
Time Domain: | |||
Time-domain analysis involves studying a signal as it varies over time. However, it might be challenging to extract features such as periodicity or noise characteristics directly from the time domain. | |||
Frequency Domain: | |||
Converting a signal into its frequency components (using techniques like the Fourier Transform) often makes it easier to identify and filter out noise, detect repeating patterns, or study signal behavior over various frequency bands. | |||
Signals: | Signals: | ||
Zeile 35: | Zeile 46: | ||
''The process of removing unwanted noise or extracting useful parts of a signal. Techniques include low-pass, high-pass, band-pass, and adaptive filters.'' | ''The process of removing unwanted noise or extracting useful parts of a signal. Techniques include low-pass, high-pass, band-pass, and adaptive filters.'' | ||
Low Pass Filters: Permit signals with frequencies lower than a cutoff frequency, useful for removing high-frequency noise. | |||
High Pass Filters: Permit frequencies higher than a cutoff frequency, often used to eliminate low-frequency drift or interference. | |||
Band Pass and Band Stop Filters: Focus on specific frequency bands. | |||
cutoff frquency: The cutoff frequency, or cutoff, determines where the signal is cut off. If a signal contains frequencies that range from 500Hz up to 8000 Hz and the cutoff frequency is set at 4000 Hz, frequencies above 4000 Hz are filtered for example in the case of low pass filters. | |||
== Task == | == Task == |
Version vom 10. April 2025, 16:06 Uhr
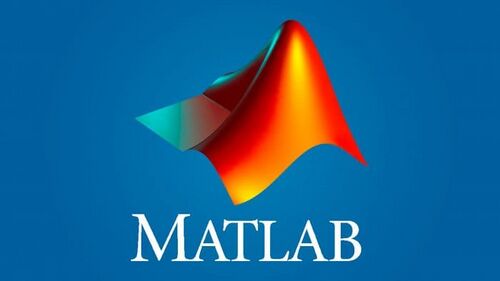
Autor: | Moye Nyuysoni Glein Perry |
Art: | Project Work |
Starttermin: | 14.11.2024 |
Abgabetermin: | 31.03.2025 |
Betreuer: | Prof. Dr.-Ing. Schneider |
MindMap
Introductiong
Signal processing has traditionally focused on analyzing, filtering, and interpreting signals in both the time and frequency domains. With the integration of AI, especially deep learning, the possibilities expand to include noise reduction and more. It is essential in many modern applications such as communications, audio and image processing, biomedical signal analysis, and control systems.
Traditionally, classical algorithms have been taught to solve engineering problems. This project solves teaching problems using artificial intelligence and compares these classical algorithms.
Signal Processing Fundamentals
Time Domain vs. Frequency Domain
Time Domain: Time-domain analysis involves studying a signal as it varies over time. However, it might be challenging to extract features such as periodicity or noise characteristics directly from the time domain.
Frequency Domain: Converting a signal into its frequency components (using techniques like the Fourier Transform) often makes it easier to identify and filter out noise, detect repeating patterns, or study signal behavior over various frequency bands.
Signals:
A signal is any piece of information that changes over time or space and can be measured. It may be continuous (analog) or discrete (digital).
Transforms:
Techniques like the Fourier Transform and the Discrete Wavelet Transform decompose signals into constituent frequency (or time-frequency) components for easier analysis.
Filtering:
The process of removing unwanted noise or extracting useful parts of a signal. Techniques include low-pass, high-pass, band-pass, and adaptive filters.
Low Pass Filters: Permit signals with frequencies lower than a cutoff frequency, useful for removing high-frequency noise.
High Pass Filters: Permit frequencies higher than a cutoff frequency, often used to eliminate low-frequency drift or interference.
Band Pass and Band Stop Filters: Focus on specific frequency bands.
cutoff frquency: The cutoff frequency, or cutoff, determines where the signal is cut off. If a signal contains frequencies that range from 500Hz up to 8000 Hz and the cutoff frequency is set at 4000 Hz, frequencies above 4000 Hz are filtered for example in the case of low pass filters.
Task
- Familiarization with MATLAB® AI toolboxes
- Researching practical application examples that can be converted to AI
- Implementation of selected examples
- Identify the advantages and disadvantages of AI compared to conventional data processing
- Discussion of the results
- Test and scientific documentation
- Providing MATLAB®-examples as wiki articles
Anforderungen
Das Projekt erfordert Vorwissen in einigen aber nicht allen nachfolgenden Themengebieten. Sollten Sie die Anforderungen nicht erfüllen, kann die Aufgabenstellung mit Blick auf Ihre Vorkenntnisse individuell angepasst werden.
- MATLAB®/Simulink
- Machine Learning/Deep Learning
- Digitale Signalverarbeitung
- Deep Learning for Image Processing
Anforderungen an die wissenschaftliche Arbeit
- Wissenschaftliche Vorgehensweise (Projektplan, etc.), nützlicher Artikel: Gantt Diagramm erstellen
- Wöchentlicher Fortschrittsberichte (informativ), aktualisieren Sie das Besprechungsprotokoll im Gespräch mit Prof. Schneider
- Projektvorstellung im Wiki
- Tägliche Sicherung der Arbeitsergebnisse in SVN
- Tägliche Dokumentation der geleisteten Arbeitsstunden
- Studentische Arbeiten bei Prof. Schneider
- Anforderungen an eine wissenschaftlich Arbeit
Image Processing
Image processing refers to the use of algorithms and computational techniques to analyse, enhance, and manipulate images. It involves altering or improving images using various methods and tools. The main aim of image processing is to improve image quality. Whether it’s enhancing contrast, adjusting colours, or smoothing edges, the focus is on making the image more visually appealing or suitable for further use. It’s about transforming the raw image into a refined version of itself.
Sample Task: Object Detection Identify in an Image
- Classical Method: Circular Objects Detection
- results:
- AI based method: Detect people, cats and dogs in message using YOLO model.
- Results
References
https://support.apple.com/guide/logicpro-ipad/cutoff-frequency-lpip5e73bf83/ipados
Repository
URL: https://svn.hshl.de/svn/MATLAB_Vorkurs/trunk/Signalverarbeitung_mit_Kuenstlicher_Intelligenz
→ zurück zum Hauptartikel: Studentische Arbeiten