Lane Keeping with AI: Unterschied zwischen den Versionen
(26 dazwischenliegende Versionen desselben Benutzers werden nicht angezeigt) | |||
Zeile 22: | Zeile 22: | ||
These methods are compared using a morphological (Zwicky) box framework that considers various design parameters. Signal processing plays a key role in preprocessing the visual data and augmenting the training dataset. | These methods are compared using a morphological (Zwicky) box framework that considers various design parameters. Signal processing plays a key role in preprocessing the visual data and augmenting the training dataset. | ||
Lane-keeping refers to the ability of a vehicle to detect and maintain its position within a designated lane on the road. It is a critical function for autonomous driving, helping to enhance road safety and reduce driver workload.Our target location is the mechatronics lab | Lane-keeping refers to the ability of a vehicle to detect and maintain its position within a designated lane on the road. It is a critical function for autonomous driving, helping to enhance road safety and reduce driver workload. Our target location is the mechatronics lab at HSHl. below are videos recorded using videos from a [[JetRacer: Autonomous Driving, Obstacle Detection and Avoidance using AI with MATLAB|JetRacer]] | ||
=== Visualized Data of Sample01 === | |||
[[Datei:Rundkurs03.avi.mp4|300px|Frameless|centered|alternativtext=Rundkurs03 output video |Rundkurs03 ]] | |||
=== Visualized Data of Sample02 === | |||
[[Datei:Rundkurs04.avi.mp4|300px|frameless|centered|alternativtext=Rundkurs03 output video |Rundkurs03 ]] | |||
Fig 2. Sample Input Data | |||
== System Requirements == | |||
The lane-keeping system must fulfill the following requirements: | |||
'''Autonomous Operation:''' maintain the vehicle in the right lane. | |||
''' Real-Time Processing:''' Process video data in real time to generate accurate steering commands. | |||
'''Data Synchronization:''' Synchronize steering angle measurements with video frames. | |||
'''Robustness:''' Handle varying lighting conditions, noise, and occlusions using signal processing techniques. | |||
'''Scalability & Adaptability:''' Provide flexibility to incorporate different AI training strategies (from-scratch CNN vs. transfer learning). | |||
'''Visualization & Analysis:''' Use MATLAB® scripts (e.g., [https://svn.hshl.de/svn/MATLAB_Vorkurs/trunk/Signalverarbeitung_mit_Kuenstlicher_Intelligenz/mFiles/LaneKeeping/zeigeMessdaten.m zeigeMessdaten.m]) to visualize and verify data and performance. | |||
'''Documentation & Reproducibility:''' Maintain thorough scientific documentation including code, parameters, and evaluation criteria. | |||
= Research and Methodological Approach= | |||
=== General Steps to Solve a Problem with AI === | |||
'''Define the Problem and Gather Data:''' | |||
First, you clearly define what you want to solve. In this case, the goal is to predict steering angles (a continuous value) from video frames (images). Data is gathered from files and videos stored in specific directories. | |||
'''Data Preprocessing and Augmentation:''' | |||
The collected raw data is rarely ready for input into an AI model. It needs preprocessing (such as resizing, normalization, and contrast adjustments) to standardize the inputs. If the available data is limited, data augmentation can be applied to artificially expand the dataset. Although this code doesn't explicitly call additional augmentation functions (like rotations or flips), the preprocessing step and combining frames from multiple data files serve as an implicit augmentation strategy for low input data scenarios. | |||
'''Model Selection and Architecture Design:''' | |||
After preparing the data, you choose a model architecture that suits your task. Since the aim is to predict a continuous steering value, a regression approach is used rather than classification. Regression models predict numerical outcomes and are ideal when the output is not a category but a continuous variable. | |||
'''Training the Model:''' | |||
With the network architecture defined and the data prepared, the next step is to train the network. During training, the network learns to map the input features (processed video frames) to the expected output (steering angles). | |||
'''Evaluation and Saving the Model:''' | |||
The trained model is evaluated using a validation set to track performance. Once the model trains successfully, it is saved for future use or further testing in a simulation. | |||
=== Solution Alternatives and Morphological Box=== | |||
The morphological box is used to systematically explore and compare various solutions based on design parameters and their possible values. The following table summarizes the alternatives: | |||
[[Datei:Basic Morphological Table(LK).png|500px|mini|Fig. 3: Basic Morphological Table|alternativtext=Fig. 3: Basic Morphological Table]] | |||
=== Signal Processing in Lane Detection=== | |||
Signal processing is central to the project: | |||
'''Preprocessing:''' Frames are converted to grayscale, contrast-adjusted, resized, and noise-filtered (using Gaussian filters and morphological operations) to enhance lane features. | |||
'''Data Augmentation:''' Multiple versions of each frame (e.g., flipped, brightness-adjusted) are created to increase dataset diversity and improve model generalization. | |||
'''Feature Extraction:''' Edge detection and Hough transforms (with morphological closing) are applied to detect lane markings robustly, especially in challenging scenarios such as dotted or faded lines. | |||
These techniques enhance model training, leading to more reliable lane detection. | |||
=Implementation in MATLAB®= | |||
Two main implementation strategies have been developed and compared. | |||
=== Data Acquisition and Preprocessing === | |||
Data Sources: Videos and corresponding steering angle data (from Messdaten_03.mat to Messdaten_15.mat) are used. | |||
https://svn.hshl.de/svn/MATLAB_Vorkurs/trunk/Signalverarbeitung_mit_Kuenstlicher_Intelligenz/Data/LaneKeeping/ | |||
Preprocessing Pipeline: | |||
Convert each frame to grayscale. | |||
Adjust contrast and resize frames (e.g., to 64×64 for the CNN from scratch or 224×224 for ResNet-50). | |||
Normalize steering angle data to a fixed range (e.g., [-0.2, 0.2]) and discretize for classification tasks. | |||
===CNN from Scratch using Regression=== | |||
Overview | |||
This approach builds a CNN from scratch with the following key steps: | |||
'''1. Initialization and Environment Set'''up | |||
clc; | |||
clear; | |||
close all; | |||
Purpose: | |||
Clears the command window, workspace variables, and closes all open figure windows to ensure no leftover data interferes with the upcoming execution. | |||
Why: | |||
This keeps the environment clean, which is a good practice in any development process. | |||
'''2. Reset GPU (if available)''' | |||
if gpuDeviceCount > 0 | |||
reset(gpuDevice(1)); | |||
end | |||
Purpose: | |||
Checks whether a GPU is available and, if so, resets it. | |||
Why: | |||
Resetting the GPU can help prevent memory issues from previous runs, ensuring the computation starts with a clean state—especially important when training deep neural networks where GPU resources are critical. | |||
'''3. Define Parameters and Directories''' | |||
dataDir = fullfile('..','..','..','Data','LaneKeeping'); | |||
trainedNetFolder = fullfile(pwd, 'Trained networks'); | |||
if ~exist(trainedNetFolder, 'dir') | |||
mkdir(trainedNetFolder); | |||
end | |||
outputVideoFolder = fullfile(dataDir, 'Output_Videos'); | |||
if ~exist(outputVideoFolder, 'dir') | |||
mkdir(outputVideoFolder); | |||
end | |||
Purpose: | |||
Set the file paths for data storage, saving trained networks, and output videos. | |||
Why: | |||
Organizing file directories helps manage data and results systematically. Creating folders dynamically ensures that necessary paths exist regardless of the initial state of the directory. | |||
'''4. Load Data from Files and Videos''' | |||
a. Initialize Variables and Define Preprocessing | |||
startIdx = 3; | |||
endIdx = 15; | |||
allSteeringAngles = []; | |||
allFeatures = []; % 4-D array: height x width x channels x frames | |||
% Preprocessing: convert frame to grayscale, adjust contrast, and resize to 64x64 | |||
preprocessFrame = @(frame) imresize(imadjust(rgb2gray(frame)), [64, 64]); | |||
Purpose: | |||
Initializes the range of files to process (from index 3 to 15) and prepares empty arrays to accumulate steering angles and video features. | |||
A preprocessing function is defined to convert color frames to grayscale, adjust the image contrast, and resize them to 64x64 pixels. | |||
Why: | |||
'''Data Preprocessing:''' | |||
Converting to grayscale and resizing reduces the computational load. Adjusting contrast can highlight essential features in the images. | |||
[[Datei:Sample Image(SP).png|500px|mini|Fig 4: Sample Training Data Generated]] | |||
'''Data Augmentation''': | |||
Even though the code does not explicitly use augmentation techniques like rotations or flips, the applied preprocessing ensures that the images are normalized and consistent. In scenarios with low input data, you might extend this pipeline with more augmentation (e.g., cropping, rotation, or noise addition) to expand the dataset artificially. | |||
b. Loop Through Data Files | |||
for i = startIdx:endIdx | |||
fileNumStr = sprintf('%02d', i); | |||
dataFile = fullfile(dataDir, ['Messdaten_' fileNumStr '.mat']); | |||
videoFile = fullfile(dataDir, ['Rundkurs' fileNumStr '.avi']); | |||
if ~exist(dataFile, 'file') | |||
warning('Data file %s not found.', dataFile); | |||
continue; | |||
end | |||
if ~exist(videoFile, 'file') | |||
warning('Video file %s not found.', videoFile); | |||
continue; | |||
end | |||
% Load steering angle data | |||
dataStruct = load(dataFile); | |||
if ~isfield(dataStruct, 'fSteeringAngle') | |||
warning('fSteeringAngle not found in %s', dataFile); | |||
continue; | |||
end | |||
steeringAngle = dataStruct.fSteeringAngle(:); % force column vector | |||
% Read video frames | |||
videoReader = VideoReader(videoFile); | |||
videoFeatures = []; | |||
while hasFrame(videoReader) | |||
frame = readFrame(videoReader); | |||
processedFrame = preprocessFrame(frame); | |||
videoFeatures = cat(4, videoFeatures, processedFrame); | |||
end | |||
% Use the smaller count between video frames and steering data | |||
numFrames = min(size(videoFeatures,4), length(steeringAngle)); | |||
allSteeringAngles = [allSteeringAngles; steeringAngle(1:numFrames)]; | |||
allFeatures = cat(4, allFeatures, videoFeatures(:,:,:,1:numFrames)); | |||
fprintf('Loaded %s and %s with %d frames.\n', dataFile, videoFile, numFrames); | |||
end | |||
Purpose: | |||
Iterates over a set of indexed files: | |||
Loads data files containing steering angles. | |||
Loads video files frame by frame, applying the preprocessing function to each frame. | |||
Synchronizes the number of frames with the available steering angle data (using the smaller count). | |||
Accumulates data across all files. | |||
Why: | |||
This step is essential in combining different data sources (numerical steering measurements and visual data). The synchronization ensures the model learns correctly by aligning features with their corresponding labels. | |||
'''Data Augmentation Context:''' | |||
If low data availability is an issue, additional augmentation methods could be introduced here (e.g., slight shifts or rotations of the frames). This code is structured to easily include such transformations during this loop. | |||
5. Normalize Steering Angles | |||
minAngle = min(allSteeringAngles); | |||
maxAngle = max(allSteeringAngles); | |||
if maxAngle == minAngle | |||
normalizedSteeringAngles = zeros(size(allSteeringAngles)); | |||
else | |||
normalizedSteeringAngles = (allSteeringAngles - minAngle) / (maxAngle - | |||
minAngle) * 0.4 - 0.2; | |||
end | |||
Purpose: | |||
Scales the steering angles to fall within a standardized range of [-0.2, 0.2]. | |||
Why: | |||
Normalization stabilizes and speeds up the training process by ensuring that the target values are within a similar and small range, reducing the risk of large gradient updates during regression. | |||
'''Regression vs. Classification:''' | |||
Since steering angles are continuous values, regression is the natural choice. Classification is more appropriate for discrete labels, but here, predicting an exact angle requires a regression approach to capture the full nuance of the output range. | |||
'''6. Split Data into Training and Validation Sets''' | |||
numTotalFrames = size(allFeatures, 4); | |||
numTraining = floor(0.8 * numTotalFrames); | |||
XTrain = allFeatures(:,:,:,1:numTraining); | |||
YTrain = normalizedSteeringAngles(1:numTraining); | |||
XVal = allFeatures(:,:,:,numTraining+1:end); | |||
YVal = normalizedSteeringAngles(numTraining+1:end); | |||
Purpose: | |||
Divides the data into training (80%) and validation (20%) sets. | |||
Why: | |||
This split enables the model to be trained and then validated on unseen data to ensure it generalizes well without overfitting. The validation set provides a measure of how the model might perform in real-world scenarios. | |||
'''7. Define Neural Network Architecture''' | |||
layers = [ | |||
imageInputLayer([64 64 1],'Normalization','none') | |||
convolution2dLayer(5, 64, 'Padding', 'same') | |||
batchNormalizationLayer | |||
reluLayer | |||
maxPooling2dLayer(2, 'Stride', 2) | |||
convolution2dLayer(3, 128, 'Padding', 'same') | |||
batchNormalizationLayer | |||
reluLayer | |||
maxPooling2dLayer(2, 'Stride', 2) | |||
convolution2dLayer(3, 256, 'Padding', 'same') | |||
batchNormalizationLayer | |||
reluLayer | |||
maxPooling2dLayer(2, 'Stride', 2) | |||
fullyConnectedLayer(128) | |||
dropoutLayer(0.3) | |||
fullyConnectedLayer(1) % Regression output | |||
regressionLayer | |||
]; | |||
Purpose: | |||
Builds a deep neural network for processing the 64×64 grayscale images. | |||
Layer Details: | |||
Input Layer: Takes images of size 64×64 with one channel. | |||
Convolutional Layers: Three sets of convolutional layers are used to extract visual features progressively. | |||
Batch Normalization and ReLU Layers: Normalize activations and introduce non-linearities, respectively, enhancing the training efficiency. | |||
Pooling Layers: Reduce spatial dimensions, helping the network become invariant to small translations. | |||
Fully Connected and Dropout Layers: Combine the extracted features into a single vector output and regularize the model by reducing overfitting. | |||
Final Layers: The last fully connected layer outputs a single value, appropriate for regression. The regressionLayer is used instead of a classification or softmax layer because our target (steering angle) is continuous. | |||
Why Regression: | |||
As steering values can vary continuously, using regression directly models this relationship rather than forcing the outputs into discrete categories, which could lose precision in predicting subtle changes in angle. | |||
'''8. Training Options''' | |||
options = trainingOptions('adam', ... | |||
'ExecutionEnvironment', 'gpu', ... | |||
'InitialLearnRate', 1e-4, ... | |||
'MaxEpochs', 300, ... | |||
'MiniBatchSize', 32, ... | |||
'ValidationData', {XVal, YVal}, ... | |||
'ValidationFrequency', floor(numTraining/32), ... | |||
'Plots', 'training-progress', ... | |||
'Verbose', false); | |||
Purpose: | |||
Specifies the training configuration: | |||
Optimizer: Adam is chosen for its adaptive learning capabilities. | |||
GPU Execution: Leverages GPU acceleration if available. | |||
Learning Rate, Epochs, and Batch Size: Tuned to balance training duration and performance. | |||
Validation Data & Frequency: Helps monitor the model's performance on unseen data during training. | |||
Plots and Verbosity: Provides a visual progress report without too many details cluttering the output. | |||
Why these Choices: | |||
The optimizer and parameter settings are tuned based on the dataset and problem complexity. For low data scenarios, careful settings (e.g., lower initial learning rate) help the network converge without overshooting optimal weights. | |||
'''9. Train the Network''' | |||
net = trainNetwork(XTrain, YTrain, layers, options); | |||
Purpose: | |||
Trains the neural network using the training dataset and the defined architecture. | |||
Why: | |||
This step applies backpropagation through the network layers to adjust the weights so that the predicted steering angles match the ground truth as closely as possible. The training phase is critical, as it is where the model learns from data. | |||
'''10. Save the Trained Network''' | |||
timestamp = datestr(now, 'yyyymmdd_HHMMSS'); | |||
trainedNetFile = fullfile(trainedNetFolder, ['rtrainednet_' timestamp '.mat']); | |||
save(trainedNetFile, 'net'); | |||
fprintf('Trained network saved to %s\n', trainedNetFile); | |||
Purpose: | |||
After training, the model is saved with a timestamp to uniquely identify the version. | |||
Why: | |||
Saving the model ensures that the trained parameters can be reused for prediction or further fine-tuning without having to retrain from scratch. It is a good practice to version your models, especially when iterating or comparing different experiments. | |||
===Transfer Learning with ResNet-50 and PID Control=== | |||
Overview | |||
This method leverages transfer learning using the pretrained ResNet-50 model: | |||
'''Data Preprocessing:''' | |||
Similar preprocessing (resizing to 224×224) is applied with additional lane detection computations. An improved lane deviation function employs Gaussian filtering, Canny edge detection, morphological closing, and Hough transforms to compute a steering correction. | |||
'''Data Augmentation:''' | |||
The dataset is augmented by flipping images and adjusting brightness, which is especially important for increasing robustness in the learning process. | |||
'''Network Adaptation:''' | |||
The final classification layers of ResNet-50 are replaced with new regression layers that output a continuous steering angle. | |||
'''Control Integration:''' | |||
The predicted steering angle is further refined using a PID controller (or an MPC variant), providing smoother control signals for lane keeping. | |||
'''Training and Simulation:''' | |||
The network is trained combined with our dataset, and the performance is validated by simulating the lane-keeping task with temporal smoothing of the steering command. | |||
'''Key Code Segments:''' | |||
% Remove final layers and add regression layers for steering angle prediction | |||
lgraph = removeLayers(lgraph, | |||
{'fc1000','fc1000_softmax','ClassificationLayer_fc1000'}); | |||
newFc = fullyConnectedLayer(1, 'Name', 'fcSteering'); | |||
regressionLayer = regressionLayer('Name', 'regOut'); | |||
lgraph = addLayers(lgraph, newFc); | |||
lgraph = addLayers(lgraph, regressionLayer); | |||
lgraph = connectLayers(lgraph, 'avg_pool', 'fcSteering'); | |||
lgraph = connectLayers(lgraph, 'fcSteering', 'regOut'); | |||
This snippet illustrates the network modification required for regression, transforming the classification network into one that predicts a continuous value. | |||
Additionally, a PID controller is implemented to smooth the output of the network, ensuring that the control signals are both responsive and stable. | |||
= Testing the Trained Network = | |||
'''1. Initialization and Environment Setup''' | |||
clc; | |||
clear; | |||
close all; | |||
Purpose: | |||
Clears the command window, all workspace variables, and any open figures. | |||
Why: | |||
It prevents conflicts from previous operations by ensuring that the simulation runs in a clean environment. | |||
'''2. Parameter Setup and Directory Preparation''' | |||
%% Parameters | |||
dataDir = fullfile('..','..','..','Data','LaneKeeping'); | |||
simulationDir = fullfile(pwd, 'Simulation Outputs'); | |||
errorThreshold = 0.02; | |||
if ~exist(simulationDir, 'dir') | |||
mkdir(simulationDir); | |||
end | |||
Purpose: | |||
dataDir specifies where the data (videos and associated steering angle files) is located. | |||
simulationDir determines where simulation outputs (visualizations and CSV results) will be saved. | |||
errorThreshold is set to determine the acceptable deviation between actual and predicted angles. | |||
Why: | |||
Organizing the file structure and predefining parameters lays the groundwork for reproducibility and ensures that all outputs are stored in a systematic manner. | |||
3. Video File Selection | |||
%% Select Video File | |||
videoFiles = dir(fullfile(dataDir, 'Rundkurs*.avi')); | |||
videoNames = {videoFiles.name}; | |||
[selectedIdx, tf] = listdlg('PromptString', 'Select a video file:', ... | |||
'SelectionMode', 'single', ... | |||
'ListString', videoNames); | |||
if ~tf | |||
error('No video selected.'); | |||
end | |||
selectedVideoName = videoNames{selectedIdx}; | |||
videoFile = fullfile(dataDir, selectedVideoName); | |||
videoFileIdx = sscanf(selectedVideoName, 'Rundkurs%02d.avi'); | |||
fprintf('Selected video: %s\n', selectedVideoName); | |||
Purpose: | |||
The script uses a file selection dialog to let the user choose which video to test. | |||
It then builds a complete file path and extracts the video index (to later load matching steering data). | |||
Why: | |||
User interaction ensures that the testing phase can target specific videos and datasets. Error-checking (in case no selection is made) prevents the script from proceeding without a valid input. | |||
4. Trained Network Selection | |||
%% Manually Select Trained Network | |||
[netFile, netPath] = uigetfile('*.mat', 'Select Trained Network File'); | |||
if isequal(netFile, 0) | |||
error('No network selected.'); | |||
end | |||
loaded = load(fullfile(netPath, netFile)); | |||
if ~isfield(loaded, 'net') | |||
error('Loaded file does not contain variable "net".'); | |||
end | |||
net = loaded.net; | |||
fprintf('Loaded trained network: %s\n', netFile); | |||
Purpose: | |||
Opens a dialog for the user to select a trained network file. It then loads the network. | |||
Why: | |||
This manual selection ensures that the exact model intended for testing is used. The error checks verify that the network is properly loaded and available as the variable net. | |||
'''5. Video and Steering Data Loading & Preprocessing''' | |||
%% Load and Preprocess Video | |||
videoReader = VideoReader(videoFile); | |||
preprocessFrame = @(frame) imresize(imadjust(rgb2gray(frame)), [64, 64]); | |||
allFeatures = []; | |||
frameCount = 0; | |||
dataFile = fullfile(dataDir, ['Messdaten_' sprintf('%02d', videoFileIdx) '.mat']); | |||
if ~exist(dataFile, 'file') | |||
error('Data file %s not found.', dataFile); | |||
end | |||
dataStruct = load(dataFile); | |||
if ~isfield(dataStruct, 'fSteeringAngle') | |||
error('fSteeringAngle not found in %s', dataFile); | |||
end | |||
steeringAngle = dataStruct.fSteeringAngle(:); | |||
Purpose: | |||
A VideoReader object opens the selected video. | |||
The same preprocessing function used in training (convert to grayscale, adjust contrast, and resize) is defined. | |||
Features (frames) are read into an array while counting the frames. | |||
The corresponding steering angle data is loaded from the matching data file. | |||
Why: | |||
Consistent preprocessing maintains the same data format as used during training. Ensuring the corresponding steering data is loaded allows accurate comparison later. | |||
6. Frame Extraction from the Video | |||
while hasFrame(videoReader) | |||
frame = readFrame(videoReader); | |||
processedFrame = preprocessFrame(frame); | |||
allFeatures = cat(4, allFeatures, processedFrame); | |||
frameCount = frameCount + 1; | |||
end | |||
numFrames = min(frameCount, length(steeringAngle)); | |||
allSteeringAngles = steeringAngle(1:numFrames); | |||
Purpose: | |||
Reads each frame from the video, preprocesses it, and stores it in a 4-D array. | |||
Why: | |||
The loop ensures every frame is processed in a consistent manner. The data is then truncated to the minimum of the available frames or steering data, keeping both sources synchronized. | |||
7. Normalizing Steering Angles | |||
%% Normalize Angles | |||
minAngle = min(allSteeringAngles); | |||
maxAngle = max(allSteeringAngles); | |||
normalizedSteeringAngles = (allSteeringAngles - minAngle) / (maxAngle - minAngle) | |||
* 0.4 - 0.2; | |||
Purpose: | |||
Scales the steering angles to the range [-0.2, 0.2] using min-max normalization. | |||
Why: | |||
This normalization matches the format used during training, ensuring that the network's output predictions are on the same scale as the original steering labels. | |||
'''8. Network Prediction and Error Calculation''' | |||
%% Predict with Network | |||
predictedAngles = predict(net, allFeatures); | |||
smoothPredictedAngles = smooth(predictedAngles, 15); | |||
absError = abs(smoothPredictedAngles - normalizedSteeringAngles); | |||
accuracyPercentage = sum(absError < errorThreshold) / numFrames * 100; | |||
% Determine evaluation category | |||
if accuracyPercentage > 90 | |||
evaluation = "Excellent lane keeping."; | |||
elseif accuracyPercentage > 70 | |||
evaluation = "Good lane keeping."; | |||
elseif accuracyPercentage > 50 | |||
evaluation = "Moderate lane keeping."; | |||
else | |||
evaluation = "Poor lane keeping."; | |||
end | |||
fprintf('Test Video Accuracy: %.2f%%\nAI Evaluation: %s\n', accuracyPercentage, | |||
evaluation); | |||
Purpose: | |||
Uses the loaded network to predict steering angles from the preprocessed frames. | |||
A smoothing function (with a window of 15) is applied to the predictions to reduce noise. | |||
The absolute error between the smoothed predictions and normalized ground-truth values is calculated. | |||
An accuracy percentage is computed based on how many predictions are within the predefined error threshold. | |||
A qualitative evaluation (Excellent/Good/Moderate/Poor) is then assigned based on the accuracy. | |||
Why: | |||
The error analysis and smoothing offer a realistic performance metric. The threshold approach simplifies the performance evaluation into easily understandable categories. | |||
9. Saving Detailed Results | |||
%% Prepare Output Folder | |||
timestamp = datestr(now, 'yyyy-mm-dd_HH-MM-SS'); | |||
testOutputFolder = fullfile(simulationDir, ['Test_' timestamp]); | |||
mkdir(testOutputFolder); | |||
csvFile = fullfile(testOutputFolder, 'results.csv'); | |||
writetable(table((1:numFrames)', normalizedSteeringAngles, | |||
smoothPredictedAngles(1:numFrames), absError(1:numFrames), ... | |||
'VariableNames', {'Frame', 'ActualAngle', 'PredictedAngle', 'AbsError'}), | |||
csvFile); | |||
Purpose: | |||
Creates a new folder named with a timestamp to save test results and outputs. | |||
A CSV file is generated containing per-frame results: actual angle, predicted angle, and absolute error. | |||
Why: | |||
Saving detailed results in a CSV facilitates further analysis, documentation, and comparison of performance metrics over multiple tests. | |||
'''10. Simulation Display and Video Generatio'''n | |||
%% Simulation Display and Output Video | |||
videoReaderSim = VideoReader(videoFile); | |||
outputVideo = VideoWriter(fullfile(testOutputFolder, 'simulation_output.avi')); | |||
outputVideo.FrameRate = 5; % Slowed-down playback | |||
open(outputVideo); | |||
figure('Name','Lane Keeping Simulation','Position',[100 100 1200 600]); | |||
frameIdx = 1; | |||
predictedHistory = []; | |||
actualHistory = []; | |||
while hasFrame(videoReaderSim) && frameIdx <= numFrames | |||
frame = readFrame(videoReaderSim); | |||
currentPredicted = max(-0.2, min(0.2, smoothPredictedAngles(frameIdx))); | |||
currentActual = normalizedSteeringAngles(frameIdx); | |||
predictedHistory(end+1) = currentPredicted; | |||
actualHistory(end+1) = currentActual; | |||
% Enhanced visual: arrow for direction | |||
arrowLength = 100; | |||
[h, w, ~] = size(frame); | |||
centerX = w / 2; | |||
centerY = h - 50; | |||
endX = centerX + currentPredicted * 500; | |||
endY = centerY - arrowLength; | |||
frame = insertShape(frame, 'FilledCircle', [centerX centerY 8], 'Color', 'blue', | |||
'Opacity', 1); | |||
frame = insertShape(frame, 'Line', [centerX centerY endX endY], ... | |||
'Color', 'red', 'LineWidth', 5); | |||
subplot(1,2,1); | |||
imshow(frame); | |||
title('Lane-Keeping Simulation'); | |||
subplot(1,2,2); | |||
plot(1:frameIdx, actualHistory, 'g', 'LineWidth', 2); hold on; | |||
plot(1:frameIdx, predictedHistory, 'r--', 'LineWidth', 2); hold off; | |||
legend('Actual', 'Predicted'); | |||
title('Steering Angle Tracking'); | |||
xlabel('Frame'); ylabel('Angle (Normalized)'); | |||
grid on; | |||
drawnow; | |||
% Write frame to video | |||
frameForVideo = getframe(gcf); | |||
writeVideo(outputVideo, frameForVideo); | |||
frameIdx = frameIdx + 1; | |||
end | |||
close(outputVideo); | |||
fprintf('Simulation saved to folder: %s\n', testOutputFolder); | |||
Purpose: | |||
A new VideoReader object is used to re-read the selected video for simulation purposes. | |||
An output video writer is created with a reduced frame rate to allow for slowed-down visualization. | |||
During the simulation: | |||
Each frame is annotated with visual cues: | |||
A blue circle indicates the center, and a red arrow (whose direction and length depend on the predicted steering angle) shows the predicted steering direction. | |||
A side-by-side subplot shows: | |||
The annotated frame. | |||
A plot of both actual and predicted steering angles across frames. | |||
Each annotated figure frame is captured and saved into the output video. | |||
Why: | |||
The visualization helps in qualitatively assessing how closely the AI predictions track the actual steering commands. Saving the simulation as a video allows for later review and presentation. | |||
= Results = | |||
The simulation output, which visually overlays the predicted steering direction on the original test video, provided immediate visual feedback on the system's performance. For each frame: | |||
* A blue indicator marked the center of the frame. | |||
* A red arrow, whose direction and length scaled with the predicted steering angle, graphically demonstrated the intended steering correction. | |||
* A side-by-side plot compared the smoothed predicted steering angles against the actual normalized angles over the sequence of frames | |||
== Summary of Findings == | |||
* The neural network is capable of reliably predicting steering angles that largely agree with the actual values observed from the test video. | |||
* Data normalization and preprocessing steps were crucial in achieving consistency between the training and testing environments. | |||
* The simulation visuals corroborate the numerical evaluation, showing that the predicted steering corrections are both accurate and smoothly transitioning over time. | |||
* The results offer a promising outlook for further enhancements, such as incorporating more diverse data augmentation techniques or fine-tuning the network architecture to improve performance further. | |||
===Simulation Output=== | |||
[[Datei:SimulationLK.mp4|500px|mini|Fig. 5: Simulation Lane keeping]] | |||
= Task = | = Task = | ||
Zeile 37: | Zeile 693: | ||
# Scientific documentation of every step as a wiki article with an animated gif | # Scientific documentation of every step as a wiki article with an animated gif | ||
---- | ---- | ||
→ zurück zum Hauptartikel: [[Signalverarbeitung_mit_MATLAB_und_Künstlicher_Intelligenz| Signalverarbeitung mit MATLAB und Künstlicher_Intelligenz]] | → zurück zum Hauptartikel: [[Signalverarbeitung_mit_MATLAB_und_Künstlicher_Intelligenz| Signalverarbeitung mit MATLAB und Künstlicher_Intelligenz]] |
Aktuelle Version vom 16. April 2025, 09:48 Uhr
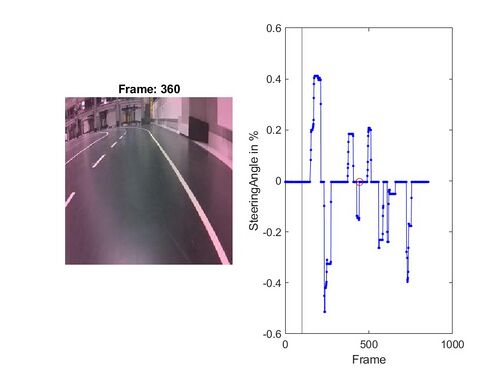
Autor: | Moye Nyuysoni Glein Perry |
Art: | Project Work |
Starttermin: | 14.11.2024 |
Abgabetermin: | 31.03.2025 |
Betreuer: | Prof. Dr.-Ing. Schneider |
Introduction
This project focuses on developing an autonomous lane-keeping system for a 1:20 scale model car equipped with a camera. The system’s goal is to train an artificial intelligence (AI) Model to drive autonomously on the track using image processing and signal processing techniques. In this work, steering angle data and video recordings are employed to train an artificial intelligence (AI) model. Two main approaches are explored:
- Training a Convolutional Neural Network (CNN) from scratch
- Using Transfer Learning (with ResNet-50 and integrated PID/MPC control)
These methods are compared using a morphological (Zwicky) box framework that considers various design parameters. Signal processing plays a key role in preprocessing the visual data and augmenting the training dataset.
Lane-keeping refers to the ability of a vehicle to detect and maintain its position within a designated lane on the road. It is a critical function for autonomous driving, helping to enhance road safety and reduce driver workload. Our target location is the mechatronics lab at HSHl. below are videos recorded using videos from a JetRacer
Visualized Data of Sample01
Visualized Data of Sample02
Fig 2. Sample Input Data
System Requirements
The lane-keeping system must fulfill the following requirements:
Autonomous Operation: maintain the vehicle in the right lane.
Real-Time Processing: Process video data in real time to generate accurate steering commands.
Data Synchronization: Synchronize steering angle measurements with video frames.
Robustness: Handle varying lighting conditions, noise, and occlusions using signal processing techniques.
Scalability & Adaptability: Provide flexibility to incorporate different AI training strategies (from-scratch CNN vs. transfer learning).
Visualization & Analysis: Use MATLAB® scripts (e.g., zeigeMessdaten.m) to visualize and verify data and performance.
Documentation & Reproducibility: Maintain thorough scientific documentation including code, parameters, and evaluation criteria.
Research and Methodological Approach
General Steps to Solve a Problem with AI
Define the Problem and Gather Data: First, you clearly define what you want to solve. In this case, the goal is to predict steering angles (a continuous value) from video frames (images). Data is gathered from files and videos stored in specific directories.
Data Preprocessing and Augmentation: The collected raw data is rarely ready for input into an AI model. It needs preprocessing (such as resizing, normalization, and contrast adjustments) to standardize the inputs. If the available data is limited, data augmentation can be applied to artificially expand the dataset. Although this code doesn't explicitly call additional augmentation functions (like rotations or flips), the preprocessing step and combining frames from multiple data files serve as an implicit augmentation strategy for low input data scenarios.
Model Selection and Architecture Design:
After preparing the data, you choose a model architecture that suits your task. Since the aim is to predict a continuous steering value, a regression approach is used rather than classification. Regression models predict numerical outcomes and are ideal when the output is not a category but a continuous variable.
Training the Model: With the network architecture defined and the data prepared, the next step is to train the network. During training, the network learns to map the input features (processed video frames) to the expected output (steering angles).
Evaluation and Saving the Model: The trained model is evaluated using a validation set to track performance. Once the model trains successfully, it is saved for future use or further testing in a simulation.
Solution Alternatives and Morphological Box
The morphological box is used to systematically explore and compare various solutions based on design parameters and their possible values. The following table summarizes the alternatives:

Signal Processing in Lane Detection
Signal processing is central to the project:
Preprocessing: Frames are converted to grayscale, contrast-adjusted, resized, and noise-filtered (using Gaussian filters and morphological operations) to enhance lane features.
Data Augmentation: Multiple versions of each frame (e.g., flipped, brightness-adjusted) are created to increase dataset diversity and improve model generalization.
Feature Extraction: Edge detection and Hough transforms (with morphological closing) are applied to detect lane markings robustly, especially in challenging scenarios such as dotted or faded lines.
These techniques enhance model training, leading to more reliable lane detection.
Implementation in MATLAB®
Two main implementation strategies have been developed and compared.
Data Acquisition and Preprocessing
Data Sources: Videos and corresponding steering angle data (from Messdaten_03.mat to Messdaten_15.mat) are used.
Preprocessing Pipeline:
Convert each frame to grayscale.
Adjust contrast and resize frames (e.g., to 64×64 for the CNN from scratch or 224×224 for ResNet-50).
Normalize steering angle data to a fixed range (e.g., [-0.2, 0.2]) and discretize for classification tasks.
CNN from Scratch using Regression
Overview This approach builds a CNN from scratch with the following key steps:
1. Initialization and Environment Setup
clc; clear; close all;
Purpose: Clears the command window, workspace variables, and closes all open figure windows to ensure no leftover data interferes with the upcoming execution.
Why: This keeps the environment clean, which is a good practice in any development process.
2. Reset GPU (if available)
if gpuDeviceCount > 0 reset(gpuDevice(1)); end
Purpose: Checks whether a GPU is available and, if so, resets it.
Why: Resetting the GPU can help prevent memory issues from previous runs, ensuring the computation starts with a clean state—especially important when training deep neural networks where GPU resources are critical.
3. Define Parameters and Directories
dataDir = fullfile('..','..','..','Data','LaneKeeping');
trainedNetFolder = fullfile(pwd, 'Trained networks'); if ~exist(trainedNetFolder, 'dir') mkdir(trainedNetFolder); end outputVideoFolder = fullfile(dataDir, 'Output_Videos'); if ~exist(outputVideoFolder, 'dir') mkdir(outputVideoFolder); end
Purpose: Set the file paths for data storage, saving trained networks, and output videos.
Why: Organizing file directories helps manage data and results systematically. Creating folders dynamically ensures that necessary paths exist regardless of the initial state of the directory.
4. Load Data from Files and Videos a. Initialize Variables and Define Preprocessing
startIdx = 3; endIdx = 15; allSteeringAngles = []; allFeatures = []; % 4-D array: height x width x channels x frames
% Preprocessing: convert frame to grayscale, adjust contrast, and resize to 64x64 preprocessFrame = @(frame) imresize(imadjust(rgb2gray(frame)), [64, 64]);
Purpose: Initializes the range of files to process (from index 3 to 15) and prepares empty arrays to accumulate steering angles and video features. A preprocessing function is defined to convert color frames to grayscale, adjust the image contrast, and resize them to 64x64 pixels.
Why:
Data Preprocessing:
Converting to grayscale and resizing reduces the computational load. Adjusting contrast can highlight essential features in the images.

Data Augmentation:
Even though the code does not explicitly use augmentation techniques like rotations or flips, the applied preprocessing ensures that the images are normalized and consistent. In scenarios with low input data, you might extend this pipeline with more augmentation (e.g., cropping, rotation, or noise addition) to expand the dataset artificially.
b. Loop Through Data Files
for i = startIdx:endIdx fileNumStr = sprintf('%02d', i); dataFile = fullfile(dataDir, ['Messdaten_' fileNumStr '.mat']); videoFile = fullfile(dataDir, ['Rundkurs' fileNumStr '.avi']); if ~exist(dataFile, 'file') warning('Data file %s not found.', dataFile); continue; end if ~exist(videoFile, 'file') warning('Video file %s not found.', videoFile); continue; end % Load steering angle data dataStruct = load(dataFile); if ~isfield(dataStruct, 'fSteeringAngle') warning('fSteeringAngle not found in %s', dataFile); continue; end steeringAngle = dataStruct.fSteeringAngle(:); % force column vector % Read video frames videoReader = VideoReader(videoFile); videoFeatures = []; while hasFrame(videoReader) frame = readFrame(videoReader); processedFrame = preprocessFrame(frame); videoFeatures = cat(4, videoFeatures, processedFrame); end % Use the smaller count between video frames and steering data numFrames = min(size(videoFeatures,4), length(steeringAngle)); allSteeringAngles = [allSteeringAngles; steeringAngle(1:numFrames)]; allFeatures = cat(4, allFeatures, videoFeatures(:,:,:,1:numFrames)); fprintf('Loaded %s and %s with %d frames.\n', dataFile, videoFile, numFrames); end
Purpose: Iterates over a set of indexed files:
Loads data files containing steering angles.
Loads video files frame by frame, applying the preprocessing function to each frame.
Synchronizes the number of frames with the available steering angle data (using the smaller count).
Accumulates data across all files.
Why: This step is essential in combining different data sources (numerical steering measurements and visual data). The synchronization ensures the model learns correctly by aligning features with their corresponding labels.
Data Augmentation Context: If low data availability is an issue, additional augmentation methods could be introduced here (e.g., slight shifts or rotations of the frames). This code is structured to easily include such transformations during this loop.
5. Normalize Steering Angles
minAngle = min(allSteeringAngles); maxAngle = max(allSteeringAngles); if maxAngle == minAngle normalizedSteeringAngles = zeros(size(allSteeringAngles)); else normalizedSteeringAngles = (allSteeringAngles - minAngle) / (maxAngle - minAngle) * 0.4 - 0.2; end
Purpose: Scales the steering angles to fall within a standardized range of [-0.2, 0.2].
Why: Normalization stabilizes and speeds up the training process by ensuring that the target values are within a similar and small range, reducing the risk of large gradient updates during regression.
Regression vs. Classification: Since steering angles are continuous values, regression is the natural choice. Classification is more appropriate for discrete labels, but here, predicting an exact angle requires a regression approach to capture the full nuance of the output range.
6. Split Data into Training and Validation Sets
numTotalFrames = size(allFeatures, 4); numTraining = floor(0.8 * numTotalFrames); XTrain = allFeatures(:,:,:,1:numTraining); YTrain = normalizedSteeringAngles(1:numTraining); XVal = allFeatures(:,:,:,numTraining+1:end); YVal = normalizedSteeringAngles(numTraining+1:end);
Purpose: Divides the data into training (80%) and validation (20%) sets.
Why: This split enables the model to be trained and then validated on unseen data to ensure it generalizes well without overfitting. The validation set provides a measure of how the model might perform in real-world scenarios.
7. Define Neural Network Architecture
layers = [ imageInputLayer([64 64 1],'Normalization','none') convolution2dLayer(5, 64, 'Padding', 'same') batchNormalizationLayer reluLayer maxPooling2dLayer(2, 'Stride', 2) convolution2dLayer(3, 128, 'Padding', 'same') batchNormalizationLayer reluLayer maxPooling2dLayer(2, 'Stride', 2) convolution2dLayer(3, 256, 'Padding', 'same') batchNormalizationLayer reluLayer maxPooling2dLayer(2, 'Stride', 2) fullyConnectedLayer(128) dropoutLayer(0.3) fullyConnectedLayer(1) % Regression output regressionLayer ];
Purpose: Builds a deep neural network for processing the 64×64 grayscale images.
Layer Details:
Input Layer: Takes images of size 64×64 with one channel.
Convolutional Layers: Three sets of convolutional layers are used to extract visual features progressively.
Batch Normalization and ReLU Layers: Normalize activations and introduce non-linearities, respectively, enhancing the training efficiency.
Pooling Layers: Reduce spatial dimensions, helping the network become invariant to small translations.
Fully Connected and Dropout Layers: Combine the extracted features into a single vector output and regularize the model by reducing overfitting.
Final Layers: The last fully connected layer outputs a single value, appropriate for regression. The regressionLayer is used instead of a classification or softmax layer because our target (steering angle) is continuous.
Why Regression: As steering values can vary continuously, using regression directly models this relationship rather than forcing the outputs into discrete categories, which could lose precision in predicting subtle changes in angle.
8. Training Options
options = trainingOptions('adam', ... 'ExecutionEnvironment', 'gpu', ... 'InitialLearnRate', 1e-4, ... 'MaxEpochs', 300, ... 'MiniBatchSize', 32, ... 'ValidationData', {XVal, YVal}, ... 'ValidationFrequency', floor(numTraining/32), ... 'Plots', 'training-progress', ... 'Verbose', false);
Purpose: Specifies the training configuration:
Optimizer: Adam is chosen for its adaptive learning capabilities.
GPU Execution: Leverages GPU acceleration if available.
Learning Rate, Epochs, and Batch Size: Tuned to balance training duration and performance.
Validation Data & Frequency: Helps monitor the model's performance on unseen data during training.
Plots and Verbosity: Provides a visual progress report without too many details cluttering the output.
Why these Choices: The optimizer and parameter settings are tuned based on the dataset and problem complexity. For low data scenarios, careful settings (e.g., lower initial learning rate) help the network converge without overshooting optimal weights.
9. Train the Network
net = trainNetwork(XTrain, YTrain, layers, options);
Purpose: Trains the neural network using the training dataset and the defined architecture.
Why: This step applies backpropagation through the network layers to adjust the weights so that the predicted steering angles match the ground truth as closely as possible. The training phase is critical, as it is where the model learns from data.
10. Save the Trained Network
timestamp = datestr(now, 'yyyymmdd_HHMMSS'); trainedNetFile = fullfile(trainedNetFolder, ['rtrainednet_' timestamp '.mat']); save(trainedNetFile, 'net'); fprintf('Trained network saved to %s\n', trainedNetFile);
Purpose: After training, the model is saved with a timestamp to uniquely identify the version.
Why: Saving the model ensures that the trained parameters can be reused for prediction or further fine-tuning without having to retrain from scratch. It is a good practice to version your models, especially when iterating or comparing different experiments.
Transfer Learning with ResNet-50 and PID Control
Overview This method leverages transfer learning using the pretrained ResNet-50 model:
Data Preprocessing: Similar preprocessing (resizing to 224×224) is applied with additional lane detection computations. An improved lane deviation function employs Gaussian filtering, Canny edge detection, morphological closing, and Hough transforms to compute a steering correction.
Data Augmentation: The dataset is augmented by flipping images and adjusting brightness, which is especially important for increasing robustness in the learning process.
Network Adaptation: The final classification layers of ResNet-50 are replaced with new regression layers that output a continuous steering angle.
Control Integration: The predicted steering angle is further refined using a PID controller (or an MPC variant), providing smoother control signals for lane keeping.
Training and Simulation: The network is trained combined with our dataset, and the performance is validated by simulating the lane-keeping task with temporal smoothing of the steering command.
Key Code Segments:
% Remove final layers and add regression layers for steering angle prediction lgraph = removeLayers(lgraph, {'fc1000','fc1000_softmax','ClassificationLayer_fc1000'}); newFc = fullyConnectedLayer(1, 'Name', 'fcSteering'); regressionLayer = regressionLayer('Name', 'regOut'); lgraph = addLayers(lgraph, newFc); lgraph = addLayers(lgraph, regressionLayer); lgraph = connectLayers(lgraph, 'avg_pool', 'fcSteering'); lgraph = connectLayers(lgraph, 'fcSteering', 'regOut');
This snippet illustrates the network modification required for regression, transforming the classification network into one that predicts a continuous value.
Additionally, a PID controller is implemented to smooth the output of the network, ensuring that the control signals are both responsive and stable.
Testing the Trained Network
1. Initialization and Environment Setup
clc; clear; close all;
Purpose: Clears the command window, all workspace variables, and any open figures.
Why: It prevents conflicts from previous operations by ensuring that the simulation runs in a clean environment.
2. Parameter Setup and Directory Preparation
%% Parameters dataDir = fullfile('..','..','..','Data','LaneKeeping'); simulationDir = fullfile(pwd, 'Simulation Outputs'); errorThreshold = 0.02; if ~exist(simulationDir, 'dir') mkdir(simulationDir); end
Purpose:
dataDir specifies where the data (videos and associated steering angle files) is located.
simulationDir determines where simulation outputs (visualizations and CSV results) will be saved.
errorThreshold is set to determine the acceptable deviation between actual and predicted angles.
Why: Organizing the file structure and predefining parameters lays the groundwork for reproducibility and ensures that all outputs are stored in a systematic manner.
3. Video File Selection
%% Select Video File videoFiles = dir(fullfile(dataDir, 'Rundkurs*.avi')); videoNames = {videoFiles.name}; [selectedIdx, tf] = listdlg('PromptString', 'Select a video file:', ... 'SelectionMode', 'single', ... 'ListString', videoNames);
if ~tf error('No video selected.'); end
selectedVideoName = videoNames{selectedIdx}; videoFile = fullfile(dataDir, selectedVideoName); videoFileIdx = sscanf(selectedVideoName, 'Rundkurs%02d.avi'); fprintf('Selected video: %s\n', selectedVideoName);
Purpose:
The script uses a file selection dialog to let the user choose which video to test.
It then builds a complete file path and extracts the video index (to later load matching steering data).
Why: User interaction ensures that the testing phase can target specific videos and datasets. Error-checking (in case no selection is made) prevents the script from proceeding without a valid input.
4. Trained Network Selection
%% Manually Select Trained Network [netFile, netPath] = uigetfile('*.mat', 'Select Trained Network File'); if isequal(netFile, 0) error('No network selected.'); end loaded = load(fullfile(netPath, netFile)); if ~isfield(loaded, 'net') error('Loaded file does not contain variable "net".'); end net = loaded.net; fprintf('Loaded trained network: %s\n', netFile);
Purpose: Opens a dialog for the user to select a trained network file. It then loads the network.
Why: This manual selection ensures that the exact model intended for testing is used. The error checks verify that the network is properly loaded and available as the variable net.
5. Video and Steering Data Loading & Preprocessing
%% Load and Preprocess Video videoReader = VideoReader(videoFile); preprocessFrame = @(frame) imresize(imadjust(rgb2gray(frame)), [64, 64]); allFeatures = []; frameCount = 0; dataFile = fullfile(dataDir, ['Messdaten_' sprintf('%02d', videoFileIdx) '.mat']); if ~exist(dataFile, 'file') error('Data file %s not found.', dataFile); end dataStruct = load(dataFile); if ~isfield(dataStruct, 'fSteeringAngle') error('fSteeringAngle not found in %s', dataFile); end steeringAngle = dataStruct.fSteeringAngle(:);
Purpose:
A VideoReader object opens the selected video.
The same preprocessing function used in training (convert to grayscale, adjust contrast, and resize) is defined.
Features (frames) are read into an array while counting the frames.
The corresponding steering angle data is loaded from the matching data file.
Why: Consistent preprocessing maintains the same data format as used during training. Ensuring the corresponding steering data is loaded allows accurate comparison later.
6. Frame Extraction from the Video
while hasFrame(videoReader) frame = readFrame(videoReader); processedFrame = preprocessFrame(frame); allFeatures = cat(4, allFeatures, processedFrame); frameCount = frameCount + 1; end
numFrames = min(frameCount, length(steeringAngle)); allSteeringAngles = steeringAngle(1:numFrames);
Purpose: Reads each frame from the video, preprocesses it, and stores it in a 4-D array.
Why: The loop ensures every frame is processed in a consistent manner. The data is then truncated to the minimum of the available frames or steering data, keeping both sources synchronized.
7. Normalizing Steering Angles
%% Normalize Angles minAngle = min(allSteeringAngles); maxAngle = max(allSteeringAngles); normalizedSteeringAngles = (allSteeringAngles - minAngle) / (maxAngle - minAngle) * 0.4 - 0.2;
Purpose: Scales the steering angles to the range [-0.2, 0.2] using min-max normalization.
Why: This normalization matches the format used during training, ensuring that the network's output predictions are on the same scale as the original steering labels.
8. Network Prediction and Error Calculation
%% Predict with Network predictedAngles = predict(net, allFeatures); smoothPredictedAngles = smooth(predictedAngles, 15); absError = abs(smoothPredictedAngles - normalizedSteeringAngles); accuracyPercentage = sum(absError < errorThreshold) / numFrames * 100;
% Determine evaluation category if accuracyPercentage > 90 evaluation = "Excellent lane keeping."; elseif accuracyPercentage > 70 evaluation = "Good lane keeping."; elseif accuracyPercentage > 50 evaluation = "Moderate lane keeping."; else evaluation = "Poor lane keeping."; end
fprintf('Test Video Accuracy: %.2f%%\nAI Evaluation: %s\n', accuracyPercentage, evaluation);
Purpose:
Uses the loaded network to predict steering angles from the preprocessed frames.
A smoothing function (with a window of 15) is applied to the predictions to reduce noise.
The absolute error between the smoothed predictions and normalized ground-truth values is calculated.
An accuracy percentage is computed based on how many predictions are within the predefined error threshold.
A qualitative evaluation (Excellent/Good/Moderate/Poor) is then assigned based on the accuracy.
Why: The error analysis and smoothing offer a realistic performance metric. The threshold approach simplifies the performance evaluation into easily understandable categories.
9. Saving Detailed Results
%% Prepare Output Folder timestamp = datestr(now, 'yyyy-mm-dd_HH-MM-SS'); testOutputFolder = fullfile(simulationDir, ['Test_' timestamp]); mkdir(testOutputFolder);
csvFile = fullfile(testOutputFolder, 'results.csv'); writetable(table((1:numFrames)', normalizedSteeringAngles, smoothPredictedAngles(1:numFrames), absError(1:numFrames), ... 'VariableNames', {'Frame', 'ActualAngle', 'PredictedAngle', 'AbsError'}), csvFile);
Purpose: Creates a new folder named with a timestamp to save test results and outputs. A CSV file is generated containing per-frame results: actual angle, predicted angle, and absolute error.
Why: Saving detailed results in a CSV facilitates further analysis, documentation, and comparison of performance metrics over multiple tests.
10. Simulation Display and Video Generation
%% Simulation Display and Output Video videoReaderSim = VideoReader(videoFile); outputVideo = VideoWriter(fullfile(testOutputFolder, 'simulation_output.avi')); outputVideo.FrameRate = 5; % Slowed-down playback open(outputVideo);
figure('Name','Lane Keeping Simulation','Position',[100 100 1200 600]); frameIdx = 1; predictedHistory = []; actualHistory = [];
while hasFrame(videoReaderSim) && frameIdx <= numFrames frame = readFrame(videoReaderSim); currentPredicted = max(-0.2, min(0.2, smoothPredictedAngles(frameIdx))); currentActual = normalizedSteeringAngles(frameIdx);
predictedHistory(end+1) = currentPredicted; actualHistory(end+1) = currentActual;
% Enhanced visual: arrow for direction arrowLength = 100; [h, w, ~] = size(frame); centerX = w / 2; centerY = h - 50;
endX = centerX + currentPredicted * 500; endY = centerY - arrowLength;
frame = insertShape(frame, 'FilledCircle', [centerX centerY 8], 'Color', 'blue', 'Opacity', 1); frame = insertShape(frame, 'Line', [centerX centerY endX endY], ... 'Color', 'red', 'LineWidth', 5);
subplot(1,2,1); imshow(frame); title('Lane-Keeping Simulation');
subplot(1,2,2); plot(1:frameIdx, actualHistory, 'g', 'LineWidth', 2); hold on; plot(1:frameIdx, predictedHistory, 'r--', 'LineWidth', 2); hold off; legend('Actual', 'Predicted'); title('Steering Angle Tracking'); xlabel('Frame'); ylabel('Angle (Normalized)'); grid on;
drawnow;
% Write frame to video frameForVideo = getframe(gcf); writeVideo(outputVideo, frameForVideo);
frameIdx = frameIdx + 1; end
close(outputVideo); fprintf('Simulation saved to folder: %s\n', testOutputFolder);
Purpose:
A new VideoReader object is used to re-read the selected video for simulation purposes.
An output video writer is created with a reduced frame rate to allow for slowed-down visualization.
During the simulation:
Each frame is annotated with visual cues:
A blue circle indicates the center, and a red arrow (whose direction and length depend on the predicted steering angle) shows the predicted steering direction.
A side-by-side subplot shows:
The annotated frame.
A plot of both actual and predicted steering angles across frames.
Each annotated figure frame is captured and saved into the output video.
Why: The visualization helps in qualitatively assessing how closely the AI predictions track the actual steering commands. Saving the simulation as a video allows for later review and presentation.
Results
The simulation output, which visually overlays the predicted steering direction on the original test video, provided immediate visual feedback on the system's performance. For each frame:
- A blue indicator marked the center of the frame.
- A red arrow, whose direction and length scaled with the predicted steering angle, graphically demonstrated the intended steering correction.
- A side-by-side plot compared the smoothed predicted steering angles against the actual normalized angles over the sequence of frames
Summary of Findings
- The neural network is capable of reliably predicting steering angles that largely agree with the actual values observed from the test video.
- Data normalization and preprocessing steps were crucial in achieving consistency between the training and testing environments.
- The simulation visuals corroborate the numerical evaluation, showing that the predicted steering corrections are both accurate and smoothly transitioning over time.
- The results offer a promising outlook for further enhancements, such as incorporating more diverse data augmentation techniques or fine-tuning the network architecture to improve performance further.
Simulation Output
Task
- Set up requirements for a lane keeping system.
- Research on solutions for the task (morphological box with technical creteria).
- Implementation in MATLAB®. Teach a AI to drive in the right lane.
- Use the Video data here.
- Use the recorded steering angle here.
- The data can be visualized with zeigeMessdaten.m (see fig. 1).
- Verify the results with Rundkurs01.avi and Rundkurs02.avi.
- Evaluation of the solutions using a morphological box (Zwicky box) based on technical creteria
- Discussion of the results
- Testing of the system requirements - proof of functionality
- Scientific documentation of every step as a wiki article with an animated gif
→ zurück zum Hauptartikel: Signalverarbeitung mit MATLAB und Künstlicher_Intelligenz